Horizontally Mobile: Tracking Human Activity
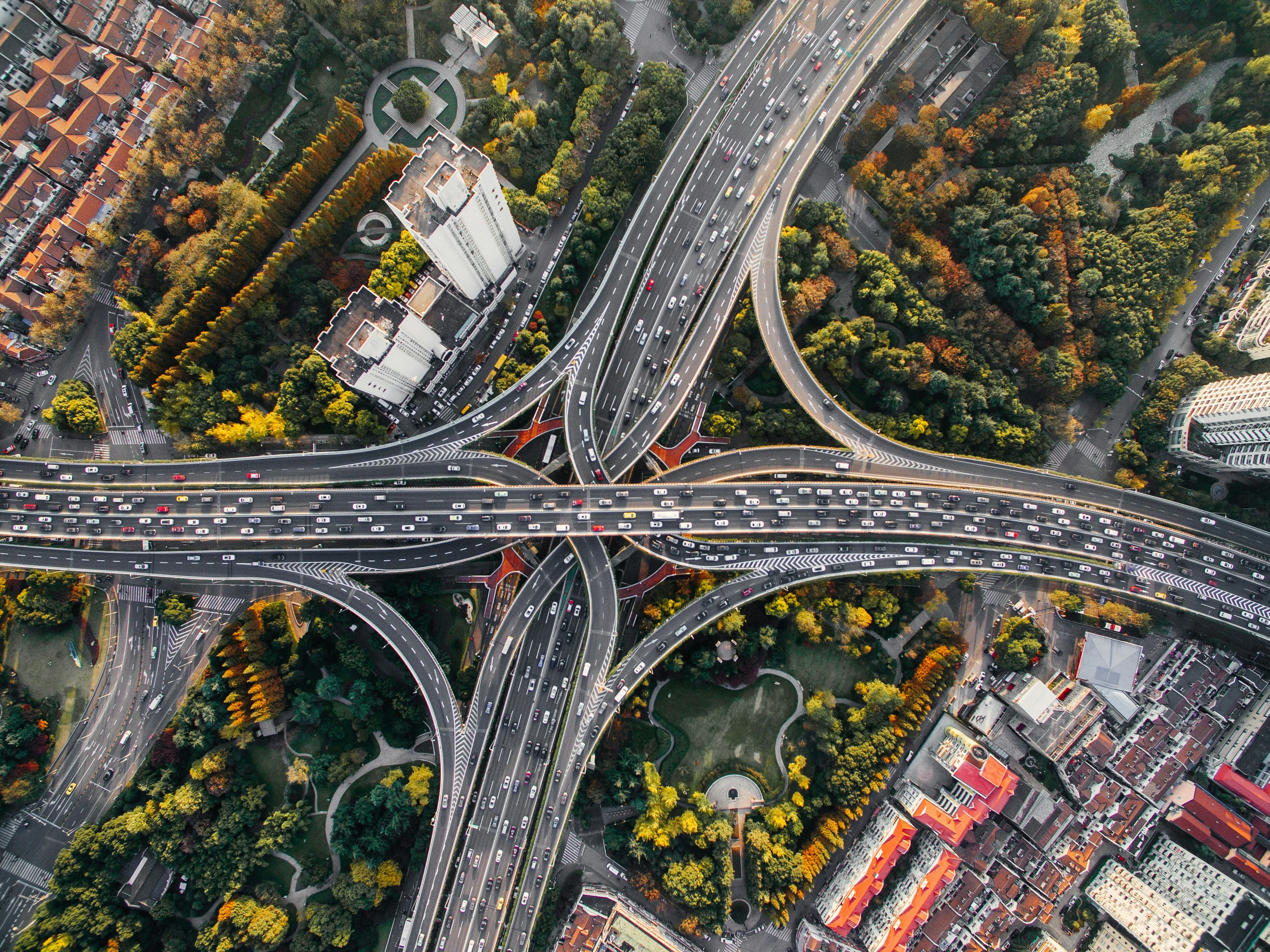
Going from mobile location services to geospatial insights.
These days, everything has an app. From weather forecasts to your favourite fast-food chain to the local gym, many of these will collect data on your location. While this seems normal, at least by today’s standards, much of this may find its way into anonymised datasets available for a price. Similarly, the location pings generated by a mobile device when using navigation software will feed into products like real-time footfall and traffic estimates. Many uses of such data are benign though some can be more onerous, especially to the increasingly privacy aware.
In this article, we’ll go through how this data is collected and what exactly we can derive from it.
What?
The location data collected is just that, plus a few extras. Termed human mobility data, in its rawest form it typically comprises no more than latitude, longitude, elevation, coordinate accuracy, timestamp, IP address, and a constant unique device identifier.
Most data providers offer a multitude of derived products and delivery methods. For the former, this can range from raw data to specialised aggregations to forecasts. In terms of delivery, ad hoc batches (via API or request and email/cloud transfer), continuous feeds, and dashboards are all options.
That said, geographical coverage varies significantly. The US is disproportionately represented in a majority of datasets, with other higher population or developed nations like the EU27 and UK observing much lower sampling rates. Similarly, temporal coverage can be tricky. A vast amount of data from disparate sources are ingested by collection and aggregation systems resulting in lengthier times taken for the dataset to contain all available data in its final verified, processed state (also called backfill).
How?
Have you ever been prompted by an app or website to enable location services? Did the request enable selection of temporary (e.g. time-limited or only when using the app) or persistent sharing? When such services are enabled for given software, the developers, administrators, or supporting systems thereof are likely to have access to the location of the device that used it, when they used it (and beyond if persistent sharing is enabled). The same may also be collected through advertisements, transactions, or connections to public networks, among other methods. Such data makes its way into the hands of those that aggregate and vend human mobility data through collaboration with those that collect it.
It should be noted that raw data collected through navigation apps like Google and Apple Maps is not known to be available for any services other than their own.
Why?
Unsurprisingly, one of the most lucrative uses yet found for such data is advertising. At a rudimentary level, knowing where, when, and/or in what quantities there’s human activity is hugely beneficial in effective placement of ads. Trade, retail, and tourism find similar benefit in consumer and cross-visitation insights, site selection and trade area analysis, and estimating foot traffic. Knowing how and when an area is used can also facilitate effective investment and development for the finance, property, and urban planning markets.
Advancing these applications in an intelligent – if slightly unnerving – way, some vendors and users alike go to great lengths to quasi-deanonymize collected data. While they may never know your name (no personally identifiable information is collected), they build up a pretty good idea of your home, place of work, and favourite shops. Such information is advantageous in modelling demographics; knowing what ads to serve, where; and the kind of visitors frequenting locations.
Human mobility data finds arguably its most altruistic use in disaster and epidemic response. Post-event analysis of catastrophes, especially when compared to a pre-event snapshot or baseline, can effectively highlight impacted areas. This is useful not only in rescue and recovery but identifying property damage and business interruption. A prominent example that ties in elements of the previously mentioned de-anonymisation is approximating flooded populated areas by unexpected movements of individual devices in conjunction with terrain data. That said, resultant disruption in mobile service has the potential to produce misleading results. Furthermore, and at the risk of stating the obvious, identifying affected areas with this data requires there to be historical activity in said areas to compare against.
Maps illustrating terrain and mobility data derived flooded area estimates (left) vs. ground truth (right). Source: Yabe, T., Tusbouchi, K., & Sekimoto, Y. (2018).
Additionally, use-cases comparing human activity over time must carefully account for historic variation, mitigating noise and anomalous information to build a representative baseline. This often requires a lengthy time series of data, significant context, and effective, efficient processing.
What next?
Thought of a way your business or products might benefit from human mobility data or just want to find out more? At GeoTech, we can help you make sense and better use of both your data and the wide range of data sources available. Please get in touch with us or reach out on LinkedIn.